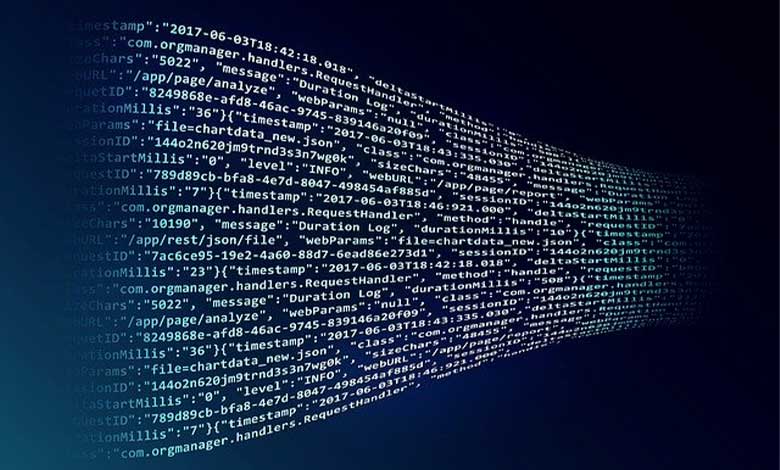
We’ve all heard the data piece.
Businesses have got to be data driven. They’ve got to connect data. They’ve got to make decisions based on that data.
It’s all true, of course. Data is the lifeblood of business today. But in my experience, the popular narrative tends to ignore the changing reality of data: the ways we connect and synthesize data, the amount and type of data generated, the compute power and techniques needed to make sense of it – all of these are constantly evolving.
Not all data is even brought into one place. Today, data can be at the edge. It can be created autonomously. It can be adversarially or otherwise intentionally manipulated. Data can beget even more data. In short, compared to a few years ago, this thing we call ‘data’ has changed in ways we are only beginning to fully understand.
And here lies a problem. With so much data – and so many different kinds of data and techniques to process it– businesses are becoming overwhelmed.
There are many risks associated with the abundance of data. With any sufficiently large amount of data, if you begin your analytic journey with preconceived notions, you’ll always find something that will support your what you believe to be true. Always. We call this confirmation bias. The idea that, if you set out to look out for something with preconceived notions, the numbers can without fail be twisted so that you can find it. Better to set out with a question than an answer.
We see this every day in the media. Stats taken out of context, or isolated to serve the agenda of whoever is presenting them. All true data does not stay true. Furthermore, without a complete understanding of the context of data, dangerous or irrational conclusions can easily arise.
The analogy I like is that we’ve gone from trying to find a needle in a haystack to finding a needle in a stack of needles. Everything drives values. “Insights” are everywhere – which makes drawing actual, meaningful inferences extremely hard.
In short, data isn’t simple. It’s messy, it’s fractured, and it’s dynamic. Many of the related challenges, such as changing regulation regarding permissible use, incomplete or altered data, inappropriate methods are presenting all at once.
Making connections
Having the data, even if we address all of the associated challenges is only the beginning. To answer meaningful questions, we must be able to connect data.
Artificial intelligence methods are an emerging capability to connect data. For example, supervised machine learning allows us to train algorithms to find patterns or relationships in large sets of data that would otherwise be overwhelming. Combining these methods with other methods, we can extrapolate into future scenarios and make meaningful inferences.
Broadly speaking, this approach works fine. But there are assumptions attached: for example, the assumption the data we curate hasn’t been manipulated, or that the conditions of the past will continue into sufficiently in the future to provide a basis of extrapolation.
Unfortunately, these assumptions don’t always turn out to be true. Especially in historically unprecedented times, or in the context of highly disruptive events.
Without a doubt, we are living through turbulent times. Brexit, Covid-19, climate change – from practically any perspective, the business landscape of today looks completely different to yesterday. There are obvious concerns associated with modelling the future based on what happened in the past.
The modern business climate is disrupted, and constantly changing due to the impact of multiple simultaneous factors. The unqualified assumption that patterns in yesterday’s data can help you draw valid inferences about tomorrow is dangerous in many ways. It is precisely that state of constant disruption that is presenting challenges to connecting data – amplified by the sheer volume and rate of change of today’s information – which mean it is increasingly difficult to draw insights.
Anomalies within anomalies
Data in today’s business enterprise is often chaotic and incomplete. If we want to get anywhere, we need a smart way to make sense of it.
At Dun & Bradstreet, there are a number of ways we do that, one of the most promising approaches relates to our focus on data anomalies.
Let’s take fraud as a concrete example.
Imagine a scenario involving commercial fraud. Simple supervised learning, looking for evidence of prior patterns of fraud is simply not sufficient. The best fraudsters tend to change their behaviour when they suspect they’ve been detected (something called an ‘observer effect’). Looking for patterns of new, coalescent behaviour that may represent fraud is confounding. Simply observing all “unusual” behaviour will result in many false signals because of the shear volume of changing behaviour at any given time. We need methods to sort the signal from the noise– to zoom in and out, look at the data and relationships from different perspectives. The goal is to establish a digital “fingerprint” of temporary coalescent behaviour that may represent a new type of fraud.
Once this fingerprint is established, then we can look for similar relationships with respect to other entities, or at different scale within an ecosystem.
Of course, this is just one method and one example. From projecting sales to working out kinks in supply chains, this is the kind of scrutiny that will soon become a necessity for all companies – regardless of size or industry.
Modern business is awash in data – and those that don’t adapt will drown in information. Businesses need to be able to extract meaningful inferences from their data. And if they don’t have the expertise in-house, they should be working with a partner who does.