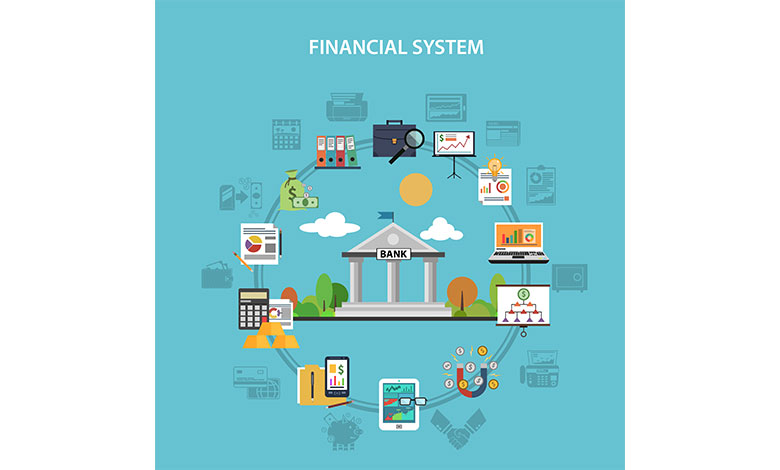
On March 24th, 2021, the United Kingdom’s Department for International Trade (DIT) and SPIN Analytics convened a Thought Leadership Roundtable to discuss how key players from France’s banking ecosystem (bankers, associations, and external advisors) are responding to COVID-19 Credit Risk challenges. The DIT and SPIN Analytics invited industry experts to share their experience, discuss the key challenges, and explore potential solutions. The roundtable was moderated by Andrew Stott, Senior Advisor to SPIN Analytics.
Essential Takeaways from the Roundtable:
*The COVID-19 pandemic introduced unprecedented levels of market turbulence and government intervention, leading to a “credit model accuracy crisis”. Banks rely heavily on models for estimating credit risk to make lending decisions and manage risk, but current approaches to modeling credit risk based on naïvely extrapolating historical trends produced dangerously inaccurate results in the crisis. Expert human judgment proved critical, yet current modeling tools are inefficient tools and left the experts overwhelmed.
*Banks have spent several years exploring the use of standardized statistical packages and machine learning tools for developing and maintaining Credit Risk Models, but the process remains manual, time-consuming (up to 6+ months per model for regulated models), labor-intensive, and costly (0.25-1million €to build, plus more for maintenance). Each Bank uses hundreds or even thousands of models, requiring support from hundreds of internal experts across multiple functions.
*Navigating through the COVID-10 crisis required exploring many different possible scenarios, so banks want to get much faster at credit risk modeling and integrating new sources of data much more quickly and efficiently. Critically, they also want to achieve this speed, efficiency, and flexibility while keeping human experts in the loop, fully in control, and spending their time on interpreting the results and applying expert judgment.
*Faster, more accurate, more forward-looking credit risk modeling remains a priority, and digitization, automation, and AI remain important components of any solution. However, the crisis has reinforced that credit risk management is about more than just historical data and statistical modeling. The next generation of tools for credit risk modeling must also integrate human judgment and credit experience.
Participants in the Roundtable included some of the biggest banks in France:
Société Générale, Crédit Agricole, October, Crédit MutuelArkea/ Financo, NatWestand other ecosystem players like EIFR, CapGemini Invent and others.
Quotes:
“The Covid crisis has shown that the ability to make forecasts on credit risks is context-dependent, which increases the importance of scenario analysis and more intensive use of analytics to better explain the decisions made in credit risk management.”
Vivien Brunel, Head of Risk and Capital Modelling, Société Générale
“Critical to improving credit risk modeling during the Covid crisis has been combining sophisticated modeling, including AI, with expert judgment. Automation is essential to support and leverage the work of experts, allowing them to focus more on tasks requiring their knowledge, experience, and judgment.”
Xavier Bocher, Head of Credit Risk Internal Models and Operational Research, Crédit Agricole
“October has achieved instant loan underwriting decisions by creating automation tools, specialized for credit risk, to use much more of the available data and accelerate the process, while leaving our experts fully in control. This gives us an advantage against established banks where credit models do not always take full advantage of the data available.”
Olivier Goy, CEO, October
“Continuing improvements in credit risk models are dependent on understanding that code is not the law and software is based on assumptions Black-box AI creates entirely new risks, with an associated tension between humans and technology. Resolving this tension requires Explainable AI in which experts remain in control and can use expert judgment to influence decisions, but also that there is trust between regulators and regulatees in integrating this new context to regulation.”
Edouard-Francois de Lenquesaing, Président European Institute for Financial Regulation
“Key success factors for financial institutions in credit risk modeling include a single data lake, improved data quality, the use of explainable AI tools to automate processes and leverage as much as possible the credit experts.”
Omar Megzari, Senior Manager at CapGemini Invent
“The Covid crisis has been a timely reminder that credit risk is about more than statistical modeling: human expertise and judgment remains more critical than ever, and by combining human expertise with automation we can go from raw data to credit models in hours or minutes instead of months.”
Julian Phillips, COO and Head of Data Science at SPIN Analytics
“The consensus from the roundtable is clear – improving data preparation and management will be key to improving the credit risk modeling process and leveraging the time of expert credit resources, and explainable AI tools will support the experts and improve their contribution, not replace them!”
Andrew Stott, formerly Head of Western Europe for Oliver Wyman and board member at BBVA, currently Senior Advisor to SPIN Analytics
The Department for International Trade (DIT) is a United Kingdom government department responsible for striking and extending trade agreements between the United Kingdom and foreign countries, as well as for encouraging foreign investment and export trade.
SPIN Analytics SPIN Analytics helps regulated banks and other credit providers improve credit risk management with its next-generation explainable AI-based platform, RISKROBOT™.
To learn more, visit http://www.SPIN-Analytics.com.